August 10, 2024: I recently completed a book chapter for the forthcoming textbook Operant Behavioral Economics, to be published by Elsevier. The chapter is titled “Quantitative models of Discounting” and the purpose is to provide a tutorial for analyzing delay discounting data using R. The book is edited by Brent Kaplan, Derek Reed, and Shawn Gilroy. Check out the arXiv link, full chapter, and data on the “Book chapter” page of my site.
June 26, 2024: Boldness-Recalibration now on CRAN! Adeline Guthrie and I were just notified that our BRcal R package has just been added to the Comprehensive R Archive Network and is now freely available. This software allows user to recalibrate and boldness-recalibrate probability forecasts for binary events. Link here: https://cran.r-project.org/web/packages/BRcal/index.html
June 6, 2024: Our paper “Flexible cost-penalized Bayesian model selection: Developing inclusion paths with an application to diagnosis of heart disease” has been published in Statistics in Medicine. The project was led by Erica Porter with Stephen Adams and myself as coauthors. The method describes a way to induce user-controlled cost penalization in Bayesian variable selection, and we introduce the idea of an inclusion path that quantifies posterior probability of a variable being non-null as a function of our cost penalization. The article is open access at the following link: https://onlinelibrary.wiley.com/doi/10.1002/sim.10113
June 6, 2024 part 2: Our paper “Thinking Inside the Bounds: Improved Error Distributions for Indifference Point Data Analysis and Simulation Via Beta Regression using Common Discounting Functions” has been published in Perspectives on Behavior Science. Mingang Kim is the lead author with Micky Koffarnus and myself as coauthors. The paper addresses deficiencies in typical regression modeling for delay discounting tasks by proposing beta regression as a method to quantify non-constant variance and to improve simulation. Of more interest to statisticians, we develop a trick that enables beta regression to accept values of zero and one without breaking. More on that later. The article is open access at the following link: https://doi.org/10.1007/s40614-024-00410-8
June 6, 2024 part 3: Recently I had the chance to catch up with Jessi Cisewski Kehe for a conversation about her work in astrostatistics. Jessi gave a killer talk about astrostats at VT some years ago, and I thought it would make for an excellent interview in Amstat News. The link to the Amstat news article is: https://magazine.amstat.org/blog/2024/05/01/starstruck-astrostatistics/
April 11, 2024: Tom Metzger and I were recently notified that our paper “Bayesian Model Selection with Latent Group-Based Effects and Variances with the R Package slgf” has been accepted for publication in The R Journal. The software we developed for this paper enables users to easily apply our method for specifying categorical factors suspected of exhibiting latent grouping, then automatically obtain Bayesian model selection-based results that assess the plausibility of these cluster structures. The paper is currently available on arXiv here: https://arxiv.org/abs/2404.06698
April 4, 2024: The open access accepted author version of our “Boldness-Recalibration for Binary Event Predictions” paper is here: https://www.tandfonline.com/doi/full/10.1080/00031305.2024.2339266?src=exp-la
April 2, 2024: Some time ago I visited the Biostatistics Branch of the National Institute of Allergy and Infectious Diseases (NIAID) in Rockville, Maryland. There were lots of cool statisticians doing lots of cool work there. I recently interviewed the Director Dean Follmann, and that conversation is now published in the current issue of Amstat News.
https://magazine.amstat.org/blog/2024/04/01/dean-follmann-niaid-interview/
March 28, 2024: I was just notified that our paper “Boldness-Recalibration for Binary Event Predictions” was accepted for publication in The American Statistician. Our boldness-recalibration method uses Bayesian model selection to enable the user to responsibly maximize boldness of their probability predictions subject to their calibration requirements. This paper was written in collaboration with Adeline Guthrie. Importantly, Adeline has developed an R package called BRcal (initial release here: https://github.com/apguthrie/BRcal) to make the method available for analysts.
March 12, 2024: I was asked to answer a few questions about statistical probability for the online magazine Bored Panda. The purpose of the article is to describe low probability events people have witnessed. They asked me a little bit about the definition of statistical probability and why I think it captures the human imagination when improbable events happen. They were good questions and it was a fun interview! Link here:https://www.boredpanda.com/lowest-possibility-events-people-personally-witnessed/?cexp_id=90923&cexp_var=55&_f=featured
February 2, 2024: Two new manuscript updates are now available on arXiv.
Boldness-Recalibration for Binary Event Predictions: https://arxiv.org/abs/2305.03780
Flexible cost-penalized Bayesian model selection: developing inclusion paths with an application to diagnosis of heart disease: https://browse.arxiv.org/abs/2305.06262
January 16, 2024: As the new year begins I thought I’d take a moment to congratulate Erica Porter on her recent graduation with a PhD from the Department of Statistics at Virginia Tech! Erica was co-advised by Dr. Marco Ferreira and I. Her dissertation studies topics in Bayesian model selection related to spatial statistics and cost-penalized feature selection. She is currently a postdoctoral fellow in the School of Mathematical and Statistical Sciences at Clemson University. Congratulations Erica!
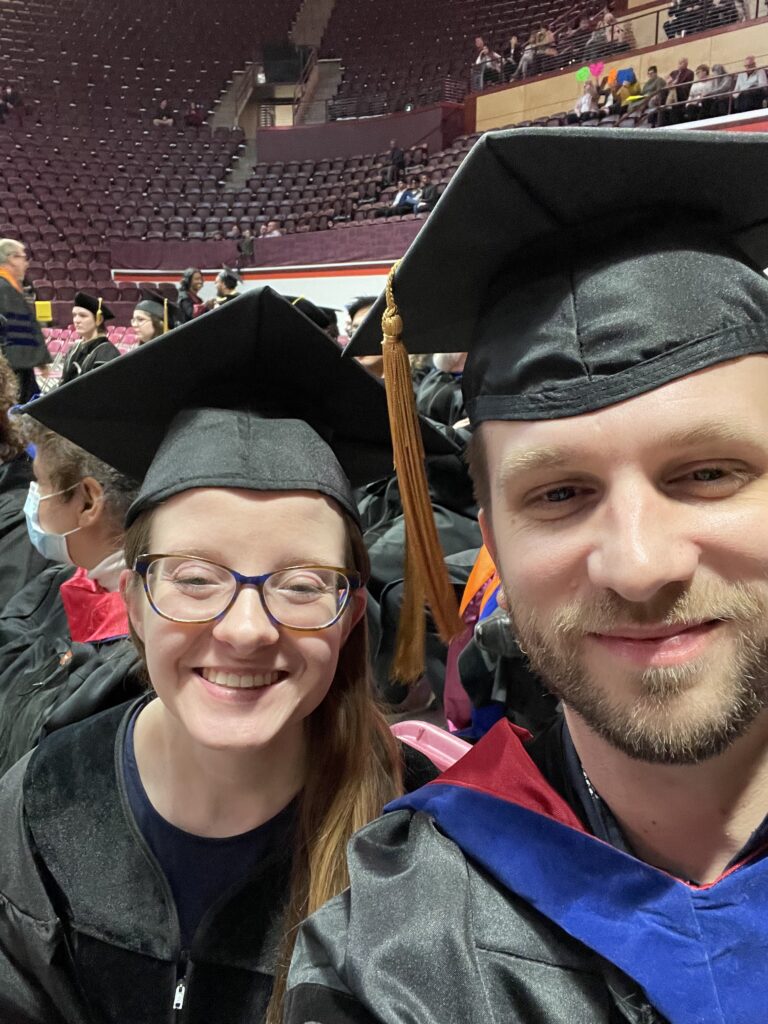
May 11, 2023: Got another new arXiv article up this week. This one was lead by Erica Porter with Stephen Adams and I as co-authors. We develop the idea of an “inclusion path” which is kind of like a lasso path, but the horizontal axis is a hyperparameter on a prior that shrinks the model, this time as a function of cost penalty. Vertical axis is posterior inclusion probability for a candidate predictor. Abstract below:
We propose a Bayesian model selection approach that allows medical practitioners to select among predictor variables while taking their respective costs into account. Medical procedures almost always incur costs in time and/or money. These costs might exceed their usefulness for modeling the outcome of interest. We develop Bayesian model selection that uses flexible model priors to penalize costly predictors a priori and select a subset of predictors useful relative to their costs. Our approach (i) gives the practitioner control over the magnitude of cost penalization, (ii) enables the prior to scale well with sample size, and (iii) enables the creation of our proposed inclusion path visualization, which can be used to make decisions about individual candidate predictors using both probabilistic and visual tools. We demonstrate the effectiveness of our inclusion path approach and the importance of being able to adjust the magnitude of the prior’s cost penalization through a dataset pertaining to heart disease diagnosis in patients at the Cleveland Clinic Foundation, where several candidate predictors with various costs were recorded for patients, and through simulated data.
May 9, 2023: I have just posted a new article written with Adeline Guthrie titled Calibration Assessment and Boldness-Recalibration for Binary Events to arXiv.
We develop a method called boldness-recalibration that enables the user to responsibly maximize boldness of their probability predictions subject to their classification accuracy. We accomplish this by developing a Bayesian model selection-based approach to assess calibration using posterior probability. Since the posterior probability is very interpretable, we are able to allow the user to, for example, prospectively choose a 95% probability of calibration, then we maximize boldness under this constraint. When accurate but overly cautious forecasters hedge too close to the base rate, our approach emboldens their predictions. If a forecaster’s accuracy is low relative to their current boldness, our same approach constricts their predictions towards the base rate to reflect limited information in their predictions. Part of the fun of this project was revisiting old analyses that produced probability predictions and using those as case studies in this article.
March 6, 2023: We were just notified that our paper Objective Bayesian Model Selection for Spatial Hierarchical Models with Intrinsic Conditional Autoregressive Priors by Erica Porter, Marco Ferreira and I was accepted for publication in Bayesian Analysis. Abstract below.
We develop Bayesian model selection via fractional Bayes factors to simultaneously assess spatial dependence and select regressors in Gaussian hierarchical models with intrinsic conditional autoregressive (ICAR) spatial random effects. Selection of covariates and spatial model structure is difficult, as spatial confounding creates a tension between fixed and spatial random effects. Researchers have commonly performed selection separately for fixed and random effects in spatial hierarchical models. Simultaneous selection methods relieve the researcher from arbitrarily fixing one of these types of effects while selecting the other. Notably, Bayesian approaches to simultaneously select covariates and spatial model structure are limited. Our use of fractional Bayes factors allows for selection of fixed effects and spatial model structure under automatic reference priors for model parameters, which obviates the need to specify hyperparameters for priors. We also show the equivalence between two ICAR specifications and derive the minimal training size for the fractional Bayes factor applied to the ICAR model under the reference prior. We perform a simulation study to assess the performance of our approach and we compare results to the Deviance Information Criterion and Widely Applicable Information Criterion. We demonstrate that our fractional Bayes factor approach assigns low posterior model probability to spatial models when data is truly independent and reliably selects the correct covariate structure with highest probability within the model space. Finally, we demonstrate our Bayesian model selection approach with applications to county-level median household income in the contiguous United States and residential crime rates in the neighborhoods of Columbus, Ohio.
September 12, 2022 I just found out that the paper I wrote with Nicole Lazar and Michael Madigan titled How to write about alternatives to classical hypothesis testing outside of the statistical literature: approximate Bayesian model selection applied to a biomechanics study was accepted for publication in Stat. Abstract below.
By now, statisticians and the broader research community are aware of the controversies surrounding traditional hypothesis testing and p values. Many alternative viewpoints and methods have been proposed, as exemplified by The American Statistician’s recent special issue themed “World beyond p<0.05.” However, it seems clear that the broader scientific effort may benefit if alternatives to classical hypothesis testing are described in venues beyond the statistical literature. This paper addresses two relevant gaps in statistical practice. First, we describe three principles statisticians and their collaborators can use to publish about alternatives to classical hypothesis testing in the literature outside of statistics. Second, we describe an existing BIC-based approximation to Bayesian model selection as a complete alternative approach to classical hypothesis testing. This approach is easy to conduct and interpret, even for analysts who do not have fully Bayesian expertise in analyzing data. Perhaps surprisingly, it does not appear that the BIC approximation has yet been described in the context of “World beyond p<0.05.” We address both gaps by describing a recent collaborative effort where we used the BIC-based techniques to publish a paper about hypothesis testing alternatives in a high-end biomechanics journal.
August 18, 2022: I just learned that my paper with Mike Madigan and Sara Arena titled “Approximate Bayesian Techniques for Statistical Model Selection and Quantifying Model Uncertainty—Application to a Gait Study” was accepted for publication in the Annals of Biomedical Engineering. This paper re-analyzes some gait data using approximate Bayesian model selection ideas based on BIC. This project induces a certain amount of discomfort, because the statistical methods we used have been well established in the statistical literature since the mid-1990s at the latest. Yet, in the early 2020s we found the opportunity to publish an exposition of this approach in a pretty darn good journal outside the field of statistics. I am glad we got the publication, but should I/we also be troubled if useful techniques that are at least 30 years old in the statistical literature seem novel to scientists in other areas? Is there an opportunity for statisticians take a more active role writing about “novel” statistical ideas in venues outside of statistics? Would that have a pretty big scientific impact? More as is develops. Abstract below.
Frequently, biomedical researchers need to choose between multiple candidate statistical models. Several techniques exist to facilitate statistical model selection including adjusted R2, hypothesis testing and p-values, information criteria, and others. One particularly useful approach that has been slow to permeate the biomedical engineering literature is the notion of posterior model probabilities. A major advantage of posterior model probabilities is that they quantify uncertainty in model selection by providing a direct, probabilistic comparison among competing models as to which is the “true” model that generated the observed data. Additionally, posterior model probabilities can be used to compute posterior inclusion probabilities which quantify the probability that individual predictors in a model are associated with the outcome in the context of all models considered given the observed data. Posterior model probabilities are typically derived from Bayesian statistical approaches which require specialized training to implement, but in this paper we describe an easy-to-compute version of posterior model probabilities and inclusion probabilities that rely on the readily-available Bayesian Information Criterion. We illustrate the utility of posterior model probabilities and inclusion probabilities by re-analyzing data from a published gait study investigating factors that predict required coefficient of friction between the shoe sole and floor while walking.
June 7, 2022: I just learned that I have been promoted to Associate Professor with tenure at Virginia Tech.
May 4, 2021: I was recently notified that my paper with Marco Ferreira and Erica Porter titled “Fast and scalable computations for Gaussian hierarchical models with intrinsic conditional autoregressive spatial random effects” has been accepted for publication in Computational Statistics and Data Analysis. Abstract below.
Fast algorithms are developed for Bayesian analysis of Gaussian hierarchical models with intrinsic conditional autoregressive (ICAR) spatial random effects. To achieve computational speed-ups, first a result is proved on the equivalence between the use of an improper CAR prior with centering on the fly and the use of a sum-zero constrained ICAR prior. This equivalence result then provides the key insight for the algorithms, which are based on rewriting the hierarchical model in the spectral domain. The two novel algorithms are the Spectral Gibbs Sampler (SGS) and the Spectral Posterior Maximizer (SPM). Both algorithms are based on one single matrix spectral decomposition computation. After this computation, the SGS and SPM algorithms scale linearly with the sample size. The SGS algorithm is preferable for smaller sample sizes, whereas the SPM algorithm is preferable for sample sizes large enough for asymptotic calculations to provide good approximations. Because the matrix spectral decomposition needs to be computed only once, the SPM algorithm has computational advantages over algorithms based on sparse matrix factorizations (which need to be computed for each value of the random effects variance parameter) in situations when many models need to be fitted. Three simulation studies are performed: the first simulation study shows improved performance in computational speed in estimation of the SGS algorithm compared to an algorithm that uses the spectral decomposition of the precision matrix; the second simulation study shows that for model selection computations with 10 regressors and sample sizes varying from 49 to 3600, when compared to the current fastest state-of-the-art algorithm implemented in the R package INLA, SPM computations are 550 to 1825 times faster; the third simulation study shows that, when compared to default INLA settings, SGS and SPM combined with reference priors provide much more adequate uncertainty quantification. Finally, the application of the novel SGS and SPM algorithms is illustrated with a spatial regression study of county-level median household income for 3108 counties in the contiguous United States in 2017.
April 9, 2021: This week I was notified that my paper with Chris Wilson titled “Predicting competitions by combining conditional logistic regression and subjective Bayes: An Academy Awards case study” was accepted for publication in the Annals of Applied Statistics. This has been a multi-year effort aimed first at predicting the winner of the Oscar for Best Picture using historical data, and later by using subjective Bayes to allow film enthusiasts to weigh their own opinions into the analysis. This is an especially meaningful one for me, because (a) it is nice to develop statistical methodology for a broad audience accessible using a data-journalism platform, and (b) because CW has been a dear friend for more than 35 years. We met in pre-school. Here we are on the first day of Kindergarten in 1987.
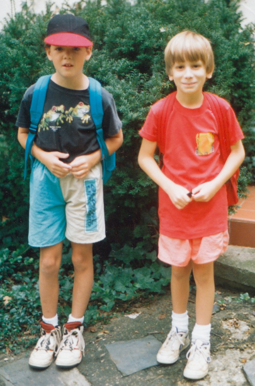
Abstract below.
Predicting the outcome of elections, sporting events, entertainment awards, and other competitions has long captured the human imagination. Such prediction is growing in sophistication in these areas, especially in the rapidly growing field of data-driven journalism intended for a general audience as the availability of historical information rapidly balloons. Providing statistical methodology to probabilistically predict competition outcomes faces two main challenges. First, a suitably general modeling approach is necessary to assign probabilities to competitors. Second, the modeling framework must be able to accommodate expert opinion, which is usually available but difficult to fully encapsulate in typical data sets. We overcome these challenges with a combined conditional logistic regression/subjective Bayes approach. To illustrate the method, we re-analyze data from a recent Time.com piece in which the authors attempted to predict the 2019 Best Picture Academy Award winner using standard logistic regression. Towards engaging and educating a broad readership, we discuss strategies to deploy the proposed method via an online application.
March 8, 2021: Last week I was notified by my co-author Tom Metzger that our R package slgf has been accepted to the Comprehensive R Archive Network. This R package extends and makes readily available the methodology in our recent Technometrics paper “Detection of latent heteroscedasticity and group-based regression effects in linear models via Bayesian model selection.” Users can specify categorical factors suspected of exhibiting latent grouping, relevant model structures of interest based on the potential for latent grouping, variance structure, (both homoscedastic and heteroscedastic), then automatic Bayesian model selection-based results are returned to the user.
March 4, 2020: I was recently notified that my paper with Tom Metzger titled “Detection of latent heteroscedasticity and group-based regression effects in linear models via Bayesian model selection” was accepted by Technometrics. The paper focuses on probabilistically detecting clusters in the levels of categorical predictors within the class of lineat models that may lead to parsimonious model contractions or expansions which reveal hidden interaction effects. The hidden group structure may also be responsible for non-constant variance. Abstract below.
Standard linear modeling approaches make potentially simplistic assumptions regarding the structure of categorical effects that may obfuscate more complex relationships governing data. For example, recent work focused on the two-way unreplicated layout has shown that hidden groupings among the levels of one categorical predictor frequently interact with the ungrouped factor. We extend the notion of a “latent grouping factor” to linear models in general. The proposed work allows researchers to determine whether an apparent grouping of the levels of a categorical predictor reveals a plausible hidden structure given the observed data. Specifically, we offer a Bayesian model selection-based approach to reveal latent group-based heteroscedasticity, regression effects, and/or interactions. Failure to account for such structures can produce misleading conclusions. Since the presence of latent group structures is frequently unknown a priori to the researcher, we use fractional Bayes factor methods and mixture g-priors to overcome lack of prior information.
September 15 2019: Today I was notified by The American Statistician that my paper with Bobby Gramacy titled “Assessing Bayes factor surfaces using interactive visualization and computer surrogate modeling” was accepted for publication. This paper suggests visualization techniques and surrogate modeling to assess sensitivity in Bayes factors as a function of hyperparameter choice. Abstract reads:
Bayesian model selection provides a natural alternative to classical hypothesis testing based on p-values. While many papers mention that Bayesian model selection can be sensitive to prior specification on parameters, there are few practical strategies to assess and report this sensitivity. This article has two goals. First, we aim to educate the broader statistical community about the extent of potential sensitivity through visualization of the Bayes factor surface. The Bayes factor surface shows the value a Bayes factor takes as a function of user-specified hyperparameters. Second, we suggest surrogate modeling via Gaussian processes to visualize the Bayes factor surface in situations where computation is expensive. We provide three examples including an interactive R shiny application that explores a simple regression problem, a hierarchical linear model selection exercise, and finally surrogate modeling via Gaussian processes to a study of the influence of outliers in empirical finance. We suggest Bayes factor surfaces are valuable for scientific reporting since they (i) increase transparency by making instability in Bayes factors easy to visualize, (ii) generalize to simple and complicated examples, and (iii) provide a path for researchers to assess the impact of prior choice on modeling decisions in a wide variety of research areas.
January 15, 2019: I was just notified that the Journal of the Experimental Analysis of Behavior has accepted our paper “An overview of Bayesian reasoning in the analysis of delay-discounting data” for inclusion in the March special issue on modern statistical practices in behavior analysis. The paper is in collaboration with Micky Koffarnus, Todd McKerhar, and Warren Bickel. Abstract reads:
Statistical inference (including interval estimation and model selection) is increasingly used in the analysis of behavioral data. As with many other fields, statistical approaches for these analyses traditionally use classical (i.e., frequentist) methods. Interpreting classical intervals and p-values correctly can be burdensome and counterintuitive. By contrast, Bayesian methods treat data, parameters, and hypotheses as random quantities and use rules of conditional probability to produce direct probabilistic statements about models and parameters given observed study data. In this work, we re-analyze two data sets using Bayesian procedures. We precede the analyses with an overview of the Bayesian paradigm. The first study re-analyzes data from a recent study of controls, heavy smokers, and individuals with alcohol and/or cocaine substance use disorder, and focuses on Bayesian hypothesis testing for covariates and interval estimation for discounting rates among various substance use disorder profiles. The second example analyzes hypothetical environmental delay-discounting data. This example focuses on using historical data to establish prior distributions for parameters while allowing subjective expert opinion to govern the prior distribution on model preference. We review the subjective nature of specifying Bayesian prior distributions but also review established methods to standardize the generation of priors and remove subjective influence while still taking advantage of the interpretive advantages of Bayesian analyses. We present the Bayesian approach as an alternative paradigm for statistical inference and discuss its strengths and weaknesses.
August 18, 2018: Today I received confirmation that my paper “Detection of hidden additivity and inference under model uncertainty for unreplicated factorial studies via Bayesian model selection and averaging” has been accepted for publication in Technometrics. This paper uses Bayesian model averaging alongside combinatoric search to conduct inference on parameters in two way unreplicated studies when the status of potential interaction effects is unknown. Abstract reads:
The two-way unreplicated layout remains a popular study design in the physical sciences. However, detection of statistical interaction and subsequent inference has been problematic in this class of designs. First, lack of replication precludes inclusion of standard interaction parameters. Second, while several restricted forms of interaction have been considered, existing approaches focus primarily on accept/reject decisions with respect to the presence of interaction. Approaches to estimate cell means and error variance are lacking when the the possibility of interaction exists. For these reasons we propose model selection and averaging-based approaches to facilitate statistical inference when the presence of interaction is uncertain. Hidden additivity, a recently proposed and intuitive form of interaction, is used to accommodate latent group-based non-additive effects. The approaches are fully Bayesian and use the Zellner-Siow formulation of the mixture g-prior. The method is illustrated on three empirical data sets and simulated data. The estimates from the model averaging approach are compared with a customized regularization approach which shrinks interaction effects toward the additive model. The study concludes that Bayesian model selection is a fruitful approach to detect hidden additivity, and model averaging allows for inference on quantities of interest under model uncertainty with respect to interaction effects within the two-way unreplicated design.
April 26, 2018: Today I learned that the College of Science at Virginia Tech decided to fund a small intramural grant application I put together with Co-PI Marco Ferreira to develop online cyberinfrastructure to enable researchers to make use of modern hierarchical modeling approached to analyze spatial data. It will be cool to embed some of our objective Bayes approaches into an accessible platform for use in research.
April 3, 2018: We learned today that Bayesian Analysis accepted our paper “Objective Bayesian Analysis for Gaussian Hierarchical Models with Intrinsic Conditional Autoregressive Priors.” This is another paper from Matt Keefe, Marco Ferreira, and I. Abstract reads:
Bayesian hierarchical models are commonly used for modeling spatially correlated areal data. However, choosing appropriate prior distributions for the parameters in these models is necessary and sometimes challenging. In particular, an intrinsic conditional autoregressive (CAR) hierarchical component is often used to account for spatial association. Vague proper prior distributions have frequently been used for this type of model, but this requires the careful selection of suitable hyperparameters. In this paper, we derive several objective priors for the Gaussian hierarchical model with an intrinsic CAR component and discuss their properties. We show that the independence Jeffreys and Jeffreys-rule priors result in improper posterior distributions, while the reference prior results in a proper posterior distribution. We present results from a simulation study that compares frequentist properties of Bayesian procedures that use several competing priors, including the derived reference prior. We demonstrate that using the reference prior results in favorable coverage, interval length, and mean squared error. Finally, we illustrate our methodology with an application to 2012 housing foreclosure rates in the 88 counties of Ohio.
March 30, 2018: Today I was notified that Spatial Statistics has accepted a manuscript submission from Matt Keefe, Marco Ferreira, and myself titled “On the Formal Specification of Sum-zero Constrained Intrinsic Conditional Autoregressive Models.” Great news! Abstract reads:
We propose a formal specification for sum-zero constrained intrinsic conditional autoregressive (ICAR) models. Our specification first projects a vector of proper conditional autoregressive spatial random effects onto a subspace where the projected vector is constrained to sum to zero, and after that takes the limit when the proper conditional autoregressive model approaches the ICAR model. As a result, we show that the sum-zero constrained ICAR model has a singular Gaussian distribution with zero mean vector and a unique covariance matrix. Previously, sum-zero constraints have typically been imposed on the vector of spatial random effects in ICAR models within a Markov chain Monte Carlo (MCMC) algorithm in what is known as centering-on-the-fly. This mathematically informal way to impose the sum-zero constraint obscures the actual joint density of the spatial random effects. By contrast, the present work elucidates a unique distribution for ICAR random effects. The explicit expressions for the resulting unique covariance matrix and density function are useful for the development of Bayesian methodology in spatial statistics which will be useful to practitioners. We illustrate the practical relevance of our results by using Bayesian model selection to jointly assess both spatial dependence and fixed effects.